Generative Art: the Relationship between
Human and Machines
By: Ryan Miles, Yundan Qiu, Mengdi Li
This project explores and discusses the relationship between human and machine in generative art; drawing machines in particular, focusing on the involvement of human in the drawing process.
Generative art is usually made by a computational system. It could be created by various forms such as a series kinetic language, a software programme, or a machine, or other procedural invention, which is set into movements with some degree of autonomy contributing to or resulting in an artwork (Tate, 2019 & Galanter, 2003).
When generative art is applied in physical spaces, with drawing machine as a representative tool, where machine is an agent, replacing human uses various drawing instruments to mark paper or another 2D medium to accurately or randomly generate visual art. However, are drawing machines creating own artworks by itself? How does human participation or control during the drawing process affect the term generative art? We have researched current drawing machines/software, and built two machines that generate artworks to explore this topic.
How to Create Generate Artworks through Drawing Machines?
Four drawing machines/software were chosen from four perspectives, and were considered and compared with background, drawing principle, predictability and reference data. We were able to compare and question the boundaries of human involvement and how this influences the creation of drawing machines.
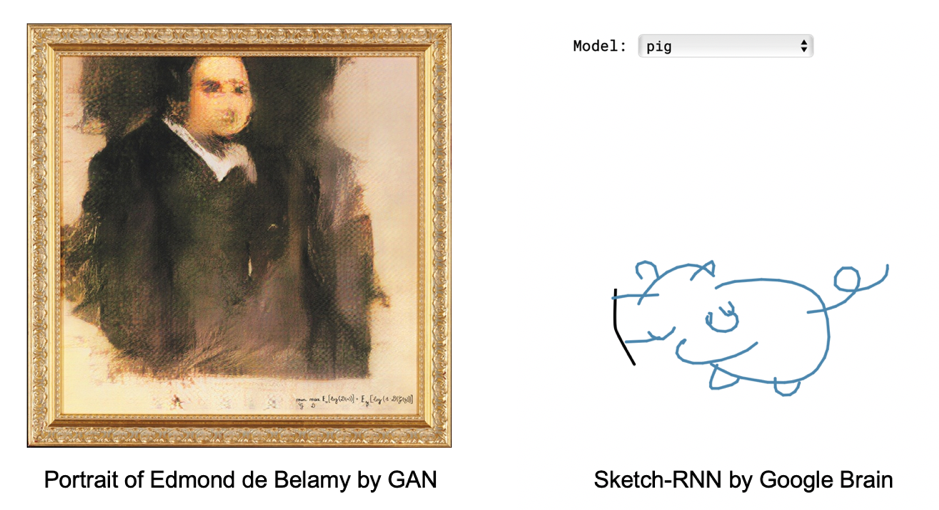
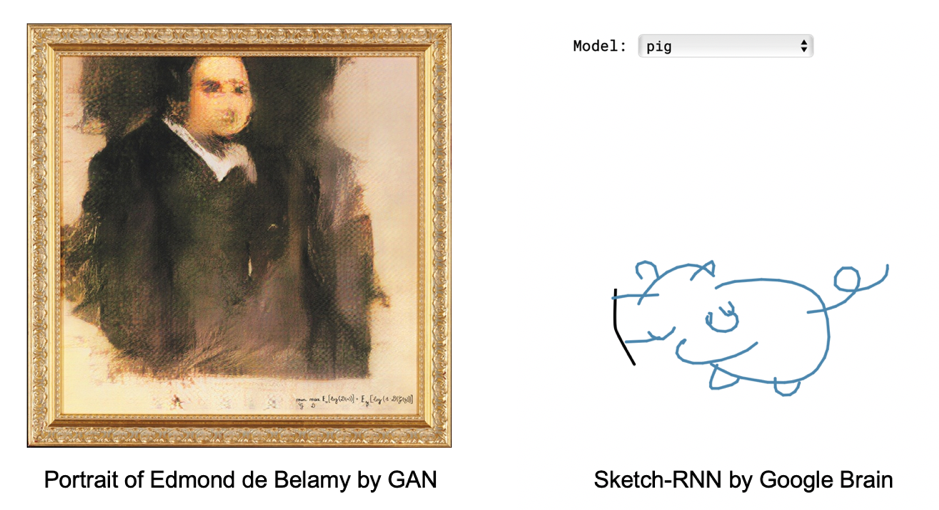
GAN(Generative Adversarial Network) and Sketch-RNN (Figure 1), working with recurrent neural network models, generating accurate and concrete patterns by learning the definition/features given by people, or human paintings/drawing actions on database (Ha and Eck, 2017). The outcomes are realistic, randomly combined with characteristics or features from database. Human participates in the early stage to input data, but have limited space to participate in the drawing process.


Both Jean Tinguely drawing machine and Machinic Trajectories, seen as Figure 2, are physical machines with abstract outcomes, which are more associated with process art, generating abstract patterns by repeating various actions. Viewers can participate and control one or more drawing stages such as drawing order, ranges or speed. basic patterns or distribution of patterns could be predicted according to preset conditions, parameters or movements, but the final visuals and content are generally unpredictable and uncontrollable.
As a result, although all machines are able to draw own original arts, they refer from preset data or instruments, which means that outcomes are partly or totally predictable. No matter how generative the artworks may seem, the creations are forever inspired from existing human painting and repeating characteristics and styles.
Can We Distinguish Machine Drawings and Human Drawings?
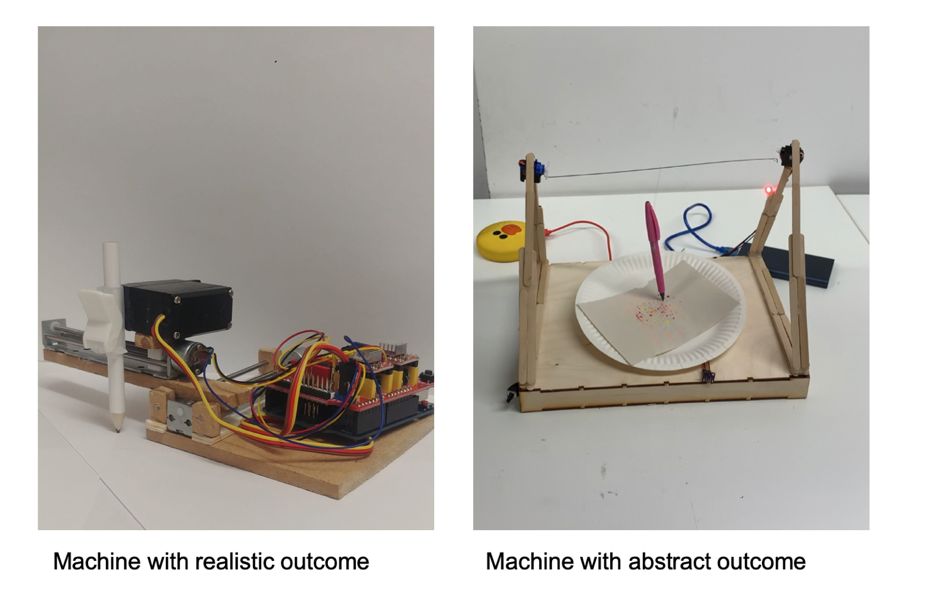
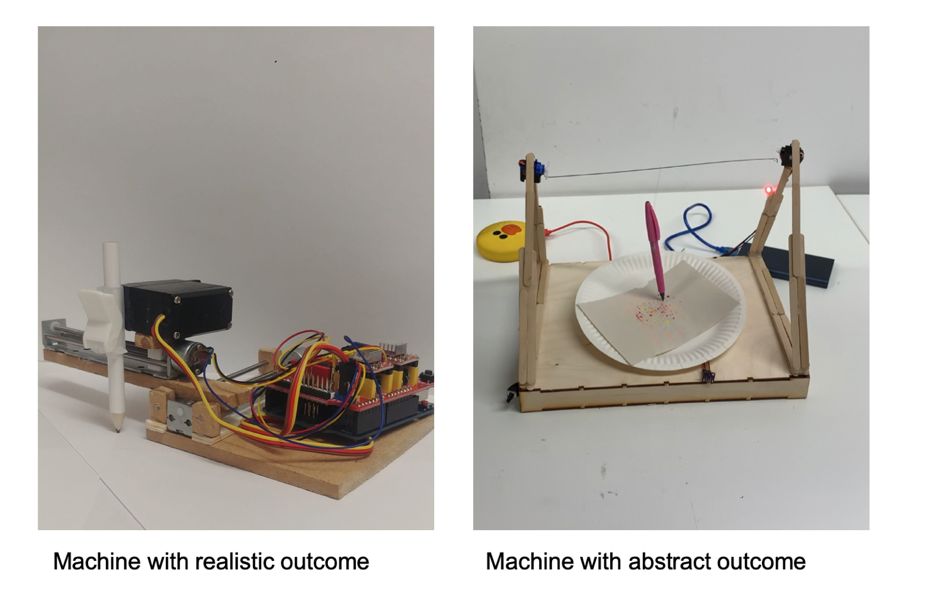
We made two drawing machines (Figure 3 and Video), one draws realistic outcomes by copying human drawings, the other one generates abstract patterns controlled by gesture sensors.
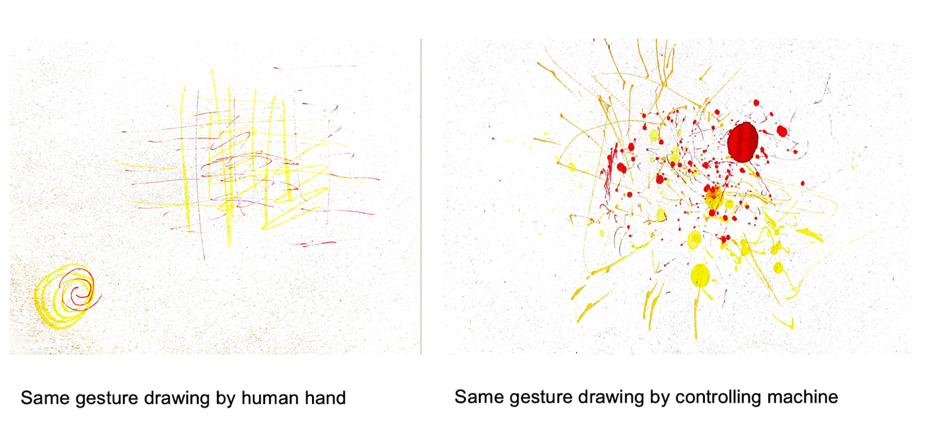
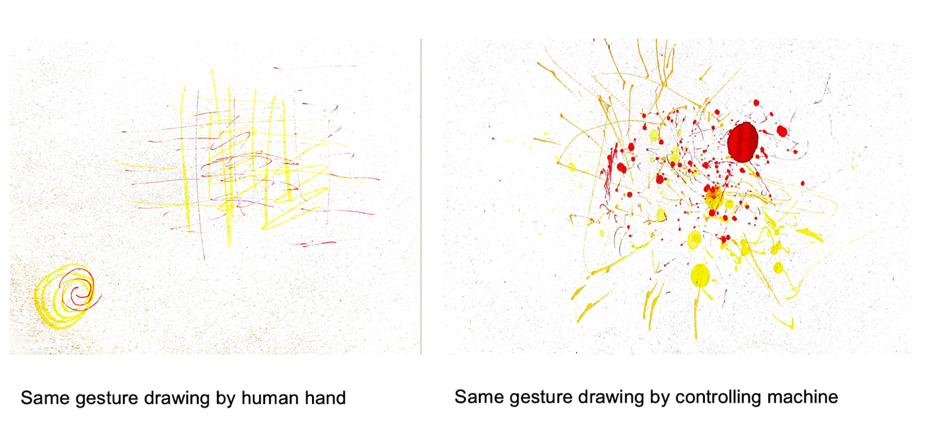
We made drawings respectively by controlling machines and the same way drawn by our hands, can be seen as Figure 4. The outcomes drawn by controlling machines are much more complicated and delicate than the patterns directly made by humans. However, in terms of the drawing characteristics such as styles or materials, it is impossible to distinguish which is machine drawing. In other words, we can draw the same pattern through other approaches.
Therefore, the drawing machine as an agent helps us to simply and efficiently generate complicated and accurate patterns, or provide human inspirations in art creation through random arrangements and combinations. It does not have human characteristics such as independent creativity. Similarly with drawing machines, when artificial intelligences identify or solve problems, they cannot break through existing human historical cognitions and achievements (Simon, 1980 & Suchman and Suchman, 2007).
Conclusion and Discussion: Human Machine Relationship and Ownership of machine Outcomes
Our original idea was to compare the results produced by the drawing machines and consider the sophistication of their outcomes. After further discussion, it seemed unethical for humans to judge or compare machine drawings in a generative art project that is considering the removal of human interaction. Instead, we decided to investigate the relationship between human and machine, focusing on the interactions between and both the predictability and reliability of the drawing machines.
Within our proposal, questions included how we can predict the outcomes and reliability of generative art and how much does the artist play a role in the creation of the artworks? We conducted primary and secondary research through the studies of theory, the histories of drawing machines and the creation of our own machines; this research indicated that the machines required some, if not solely depended on human interaction to produce results that were completely predictable or semi-predictable (Galanter, 2003). This could be due to the fact that machine lacks the capability to produce original results and interpret individual style or creativity; creating a degree of order within both the machine and the results influenced by human interaction (Galanter, 2003; Siedall, 2006; Arnheim, 2001; Marcolli, 2015).
This sparked discussion with regards to the ethics of drawing machines and who the drawing belonged to. Warren Sack considers the possibility that artists and art are at the root of software (Sack, 2019). Whilst our focus is on the physical drawing machines, many of such have integrated software to operate the machines, including our own machines. With that being said, to consider a separation between human and machine could be seen as a false statement, as there is always an element of control from the artist (Soderman and Howe, 2019). This argument is reinforced by Matt Pearson in ‘Generative Art: A Practical Guide Using Processing’, that whilst there is autonomy, the artist provides limitations and control (2011). Counteracting this, Pearson also states that there needs to be an element of surprise on the artists behalf for the artwork to remain generative (2011). Based on our primary research and creation of our own drawing machines, we received mixed results on the element of surprise. This may be due to the nature of our machines and the predictability prior to the operation of the machines.
In addition, studies on the anthropomorphism of machine were explored in relation to the ethics of drawing machines and the outcomes they produce. Whilst we respect and consider the concepts portrayed in Lucy Suchman’s ‘Figuring the Human in AI and Robotics’, we do not believe that the drawing machines we researched or created possessed human characteristics. We believe an important part of reality and possessing humanistic characteristics is for “the body [to] act as a kind of receiver for stimuli given by the world, and generator of appropriate response to it” (Suchman, 2006:230). In turn, we believe that the drawing machines should rather be considered as a tool for human expression, much like a paintbrush.
Further research then proposes questions that consider what it means to evaluate machine drawings. Who can it be evaluated by if not by humans?
Our podcast:
Reference
Arnheim, Rudolf (2001) Entropy and Art: An Essay on Disorder and Order. http://www.aakkozzll.com/pdf/arnheim.pdf (Accessed on 10/11/2019)
Chau, Christina (2014) ‘Movement and Time in the Nexus between Technological Modes with Jean Tinguely’s Kineticism’ in Arts 2014, 3, 394-406
Cohen, H. (2019). Harold Cohen Home Page. [online] Aaronshome.com. Available at: http://www.aaronshome.com/aaron/index.html [Accessed 10 Dec. 2019].
Encyclopedia Britannica. (2019). Kinetic sculpture. [online] Available at: https://www.britannica.com/art/kinetic-sculpture [Accessed 16 Dec. 2019].
Forbes.com. (2019). Council Post: GANs And Deepfakes Could Revolutionize The Fashion Industry. [online] Available at: https://www.forbes.com/sites/forbestechcouncil/2019/05/21/gans-and-deepfakes-could-revolutionize-the-fashion-industry/#1b074ace3d17 [Accessed 10 Dec. 2019].
Galanter, Philip (2003) ‘What is Generative Art’ Available at: https://pdfs.semanticscholar.org/fe29/7a3b3217824343d3ef84c9fc7eec3d8893cc.pdf [Accessed on 09/11/2019]
Lab: Understanding Complex Deep Generative Models using Interactive Visual Experimentation." IEEE Transactions on Visualization and Computer Graphics, 25(1) (VAST 2018), Jan. 2019.
Marcolli, Matilde (2015) Entropy and Art studies: The View Beyond Arnheim http://www.its.caltech.edu/~matilde/EntropyArtChapter.pdf [Accessed on 10/11/2019]
Minsuk Kahng, Nikhil Thorat, Polo Chau, Fernanda Viégas, and Martin Wattenberg. "GAN Peason, Matt (2011) Generative Art: A Practical Guide Using Processing. Manning Publications Co.
Poloclub.github.io. (2019). GAN Lab: Play with Generative Adversarial Networks in Your Browser!. [online] Available at: https://poloclub.github.io/ganlab/ [Accessed 10 Dec. 2019].
Sack, Warren (2019) The Software Arts. MIT Press 2019
Siedall, Daniel (2006) ‘Art and Failure’ in The Journal of Aesthetic Education, Vol 40, No. 2, Summer 2006.
Simmons, Seymour (2019) ‘Drawing in the Digital Age: Observations and Implications for Education’ in mdpi.com/journal/arts. Arts
Soderman, Braxton and Howe, Daniel (2019) “A Critique of Surprise in Generative Art.” Algorithmic and Generative Art, edited by Aceti, Lanfranco, Kris Paulsen, and Meredith Hoy. Leonardo Electronic Almanac 22, no. 4 (March 15, 2019).
Suchman, Lucy (2006) ‘Figuring Human in AI and Robotics’ in Human-Machine Reconfigurations. Cambridge University Press: New York.
Robotart.org. (2019). Robotart | The Robotic Art Competition. [online] Available at: https://robotart.org/ [Accessed 16 Dec. 2019].
Rolez, Anaïs (2019) ‘The Mechanical Art of Laughter’ in Arts ; Basel Vol. 8, Iss. 1, (Mar 2019)
Ha, D., & Eck, D. (2017). A Neural Representation of Sketch Drawings. ArXiv:1704.03477 [Cs, Stat]. Retrieved from http://arxiv.org/abs/1704.03477
Tate (2019) ‘Art Term: Generative Art’ available at: https://www.tate.org.uk/art/art-terms/g/generative-art [Accessed on 09/11/2019]
Suchman, L., & Suchman, L. A. (2007). Human-machine reconfigurations: Plans and situated actions. Cambridge university press.
Simon, H. A. (1980). Cognitive science: The newest science of the artificial. Cognitive science, 4(1), 33-46.