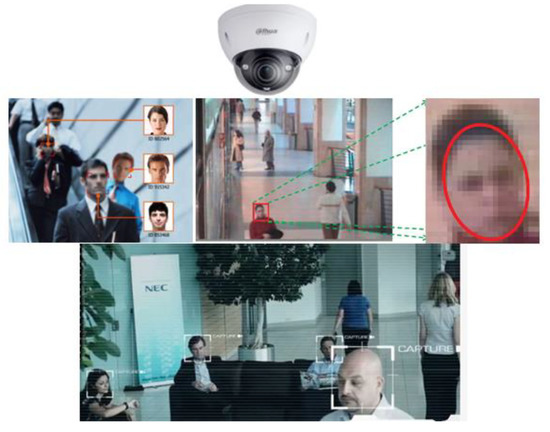
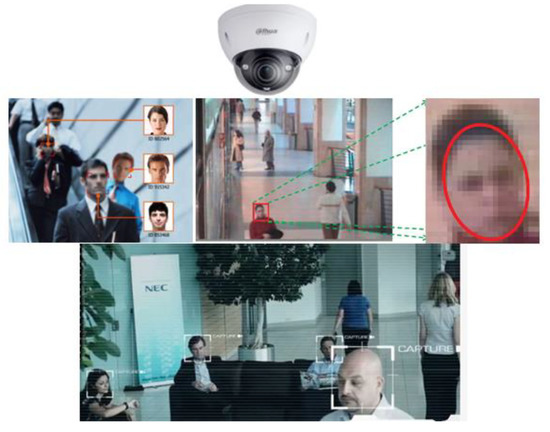
Big Brother: Racial Biases and Methods of Resistance in a world of Surveillance
Can machine learning algorithms be truly neutral? And if not, what implications do these biases have in surveillance technology?
produced by: Zoe Caudron and Timothy Lee
Introduction
Facial recognition software has emerged as one of the most active fields of research in computer vision and pattern recognition over the past few decades, and the methods of its applications are being actively utilized and explored by both private and federal institutions as biometric identifiers (Adjabi et. al., 2020). Currently, automated facial recognition requires that the machine detects the face, extracts its features to depict an accurate face normalization, and classifies it through verification or identification (Bueno, 2019). As a result, although facial recognition algorithms may seem neutral, the rules generated by the machines come from large databases of facial images that are often racially biased or not comprehensive.
Although facial recognition technology is meant to be specific, and recognize individual faces from a large pool of data, the inherent biases present in the algorithms contradict their design. Specifically, the Gender Shades project launched by the Algorithmic Justice League (AJL) exposed the divergent error rates across demographic groups and showed that the poorest accuracy was found within Black people, and particularly Black women, when compared to white men – at most with an error difference of 34.4% (Buolamwini & Gebru, 2018). It’s clear that facial recognition technology continues to be developed in a world deeply affected by racial disparities and as a result, reinforces racial discrimination. In most Western countries, Black people are more likely to be stopped and investigated by a police officer, and have their biometric information – including a face photo – entered into a bureaucratic database, which is then used by the machines to optimize their algorithms (Bacchini & Lorusso, 2019). As Ruha Benjamin aptly describes this paradox in Race after technology, “some technologies fail to see Blackness, while others render Black people hypervisible and expose them to systems of racial surveillance (Benjamin, p. 99).
Live Facial Recognition is known for the heavy racial bias it perpetuates, due to algorithms being trained on datasets lacking diversity (Fussey & Murray, 2019) . Creating fairer databases could be a way to counter the issues stemming from these biases (Lamb, 2020), but could only do so much as long as racism prevails in western countries. Could art spark difficult conversations and help highlight the urgency in legitimising the right to privacy and individual agency (Nisbet, 2004), and notably the heavier policing of marginalised communities?
In addition to decolonising training databases for major LFR softwares, there is a need to engage in a bigger conversation about the invasive rise of mass surveillance as a threat to individual freedom under a guise of “care” (Crawford & Paglen, 2019) .
By disrupting mainstream narratives around the notion of identity/identities, self, and the passive acceptance of this enforced state of surveillance, artistic practices can play a key role in resisting insidious systemic discriminations (Duggan, 2014). More specifically, art that doesn’t focus only on individual avoidance and aesthetic but brings awareness and put oppressive systems and their perpetrators under a spotlight will effectively challenge said state of surveillance (Monahan, 2015), as seen in recent mass protests that turned into worldwide performances against racial discrimination (McGarry, Erhart, Eslen-Ziya, Jenzen & Korkut, 2019). It is important to approach practices with intersectionality in mind, to start a shift from individual responsibility to collective action.
For an in-depth introduction into facial-recognition technology, algorthmic bias, topics of surveillance, and how artists are integrating these concerns into their practices, we invite you to read our longform text here.
Artefact: Exploring the accuracy of facial recognition technology on commonly used social media platforms and software
The 2020 mass protests denouncing racial violence and racism in the US, UK and Western countries in general highlighted a new danger: authorities using social media pictures to identify and target activists. Mobile facial recognition applications, formerly used for convenience and to assist in tagging a large amount of photographs, are being used to incriminate those participating in demonstrations and protest movements. Since smartphones and laptops store the time and location associated with pictures, the risks are even greater. So how well can they track and identify a face?
Our research is an expansion upon projects already undertaken by others in testing the pattern recognition capacities of Facial Recognition technology by disrupting the main features of the face that serve as anchor points for certification. CV Dazzle make-up, named after the Dazzle makeup used as camouflage by soldiers, aims to change the levels of contrast between structural components of the face - such as the bridge of the nose, the eyebrows, the cheeks, and the mouth - through pigmentation and obstruction. The idea is that these disruptions will prevent softwares from reliably identifying these facial anchor points and thus reduce the rate at which it accurately identifies a face.
Our first two testing platforms were Snapchat and Instagram - we chose these two social media platforms because they're used extensively in the general population, meaning the machine's recognition algorithms will have been trained at length and at depth. In addition, those softwares have been used and in circulation for a long period of time; as a result, users of these applications will have inevitably submitted a large database of information to these algorithms through the course of their subscription and interaction. In short, Snapchat and Instagram possess a very large community of dedicated, long-time users who actively engage with its programs and use its Facial Recognition technology for taking and sharing selfies.
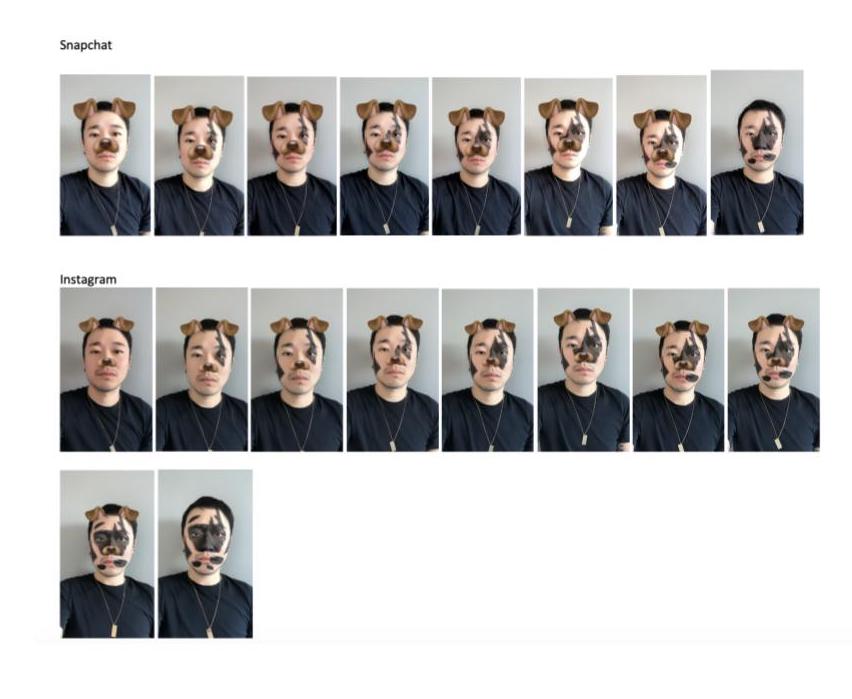
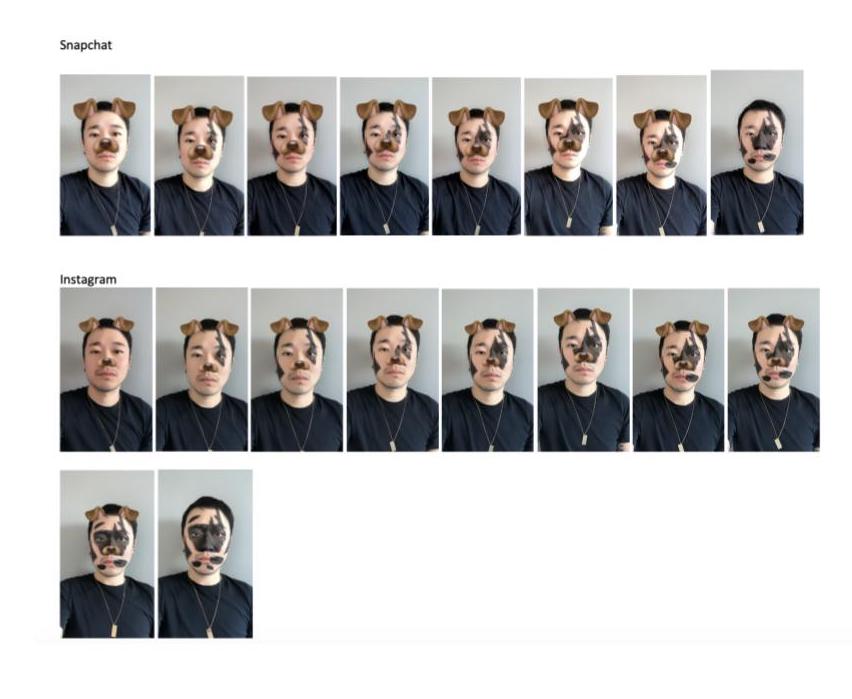
Our investigations into the limitations of Snapchat and Instagram's facial recognition software began by calibrating the applications for accuracy and consistently. We developed a working definition of a positive identification: the application must be able to reliably detect a face (and thus superimpose over the face a dog face filter) five times correctly in a row for it to be considered a true match. Once this was established, we began to add markings to our face incrementally, following our baseline rule above for true matches, until the applications were no longer able to recognize a face (the dog face filter is unsuccessful).
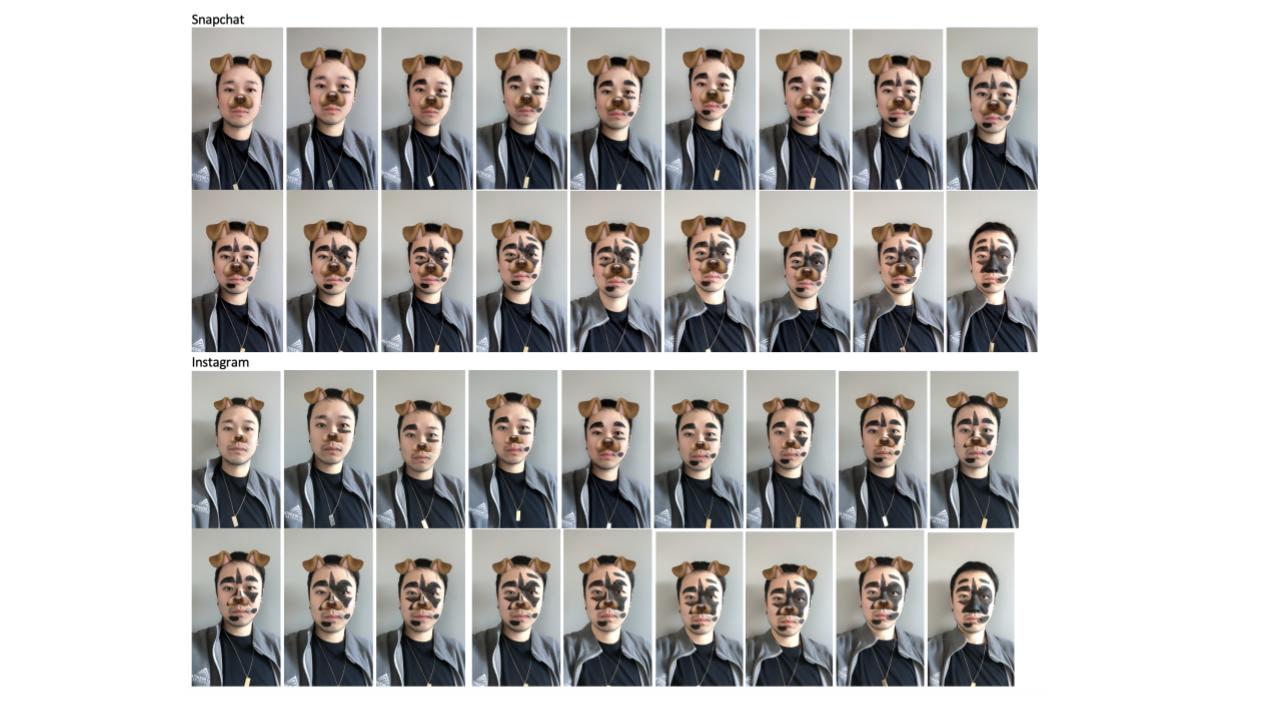
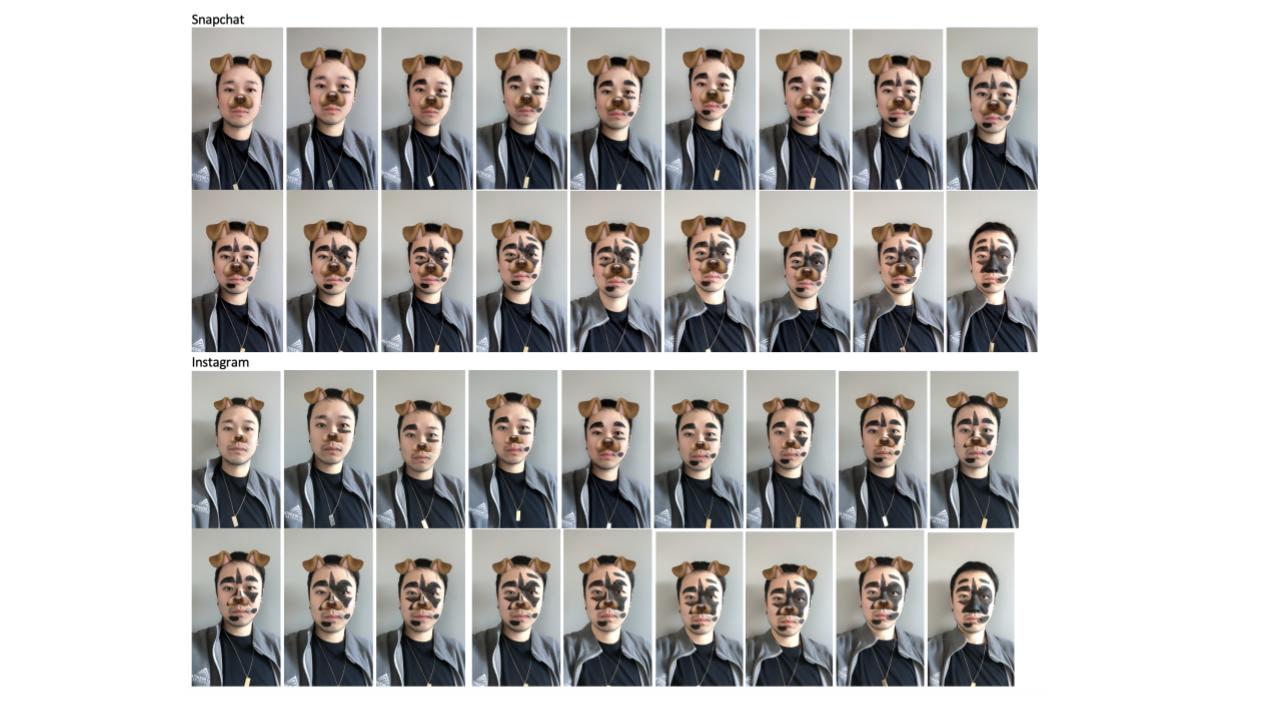
Our results were in line with many of the research done on CV Dazzle and the limitations of using makeup to combat facial recognition software. As many of the key features of the face were blackened out, we noticed the softwares increasingly have difficulty in recognizing the face. In particular, we noticed that when the T-region of the eyebrows and nose were blacked out, the facial recognition softwares of Snapchat and Instagram began to glitch and have difficulty aligning the filter with the face. This suggests that these specific structural aspects of the face are key to facial recognition software, since everyone possesses these facial features and the T-region often catches the light and casts shadows at a higher contrast. Displacing the location of the mouth or the cheekbones with make-up did not show a significant change to how our faces were recognized and certified. And in comparing the accuracy of Snapchat versus Instagram, we did not see enough of a difference to suggest that one application is better than the other in recognizing faces.
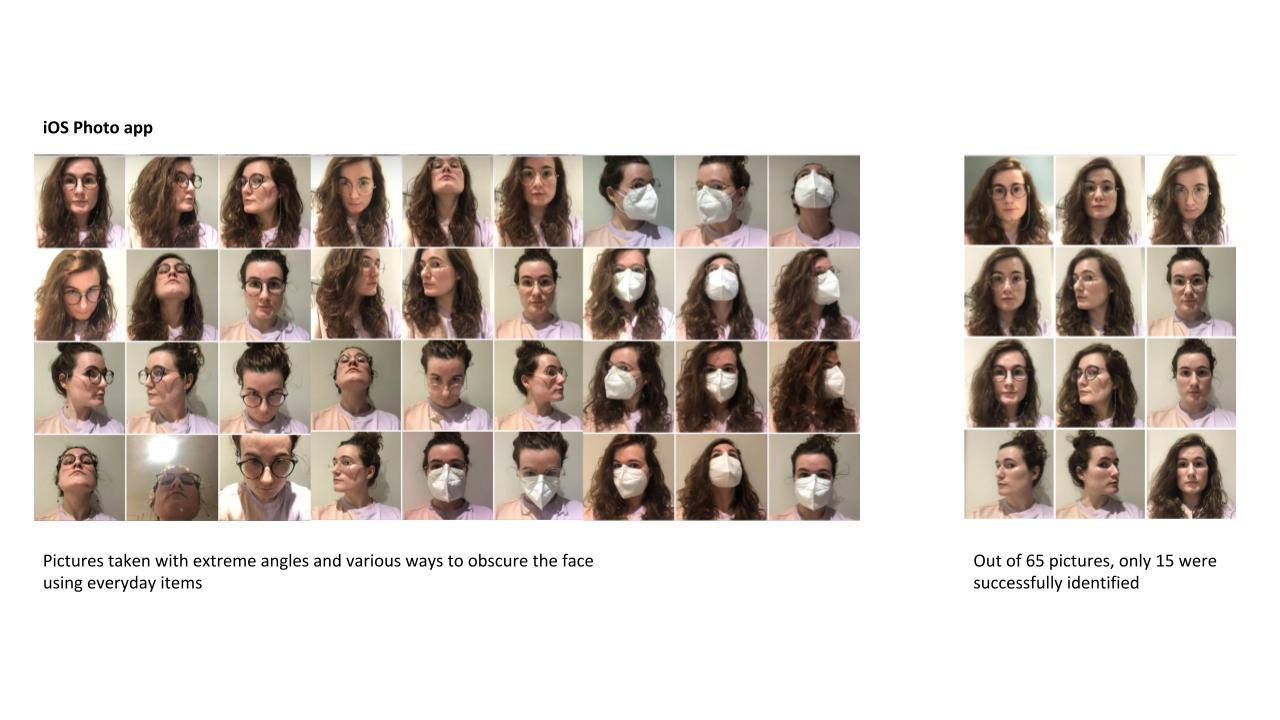
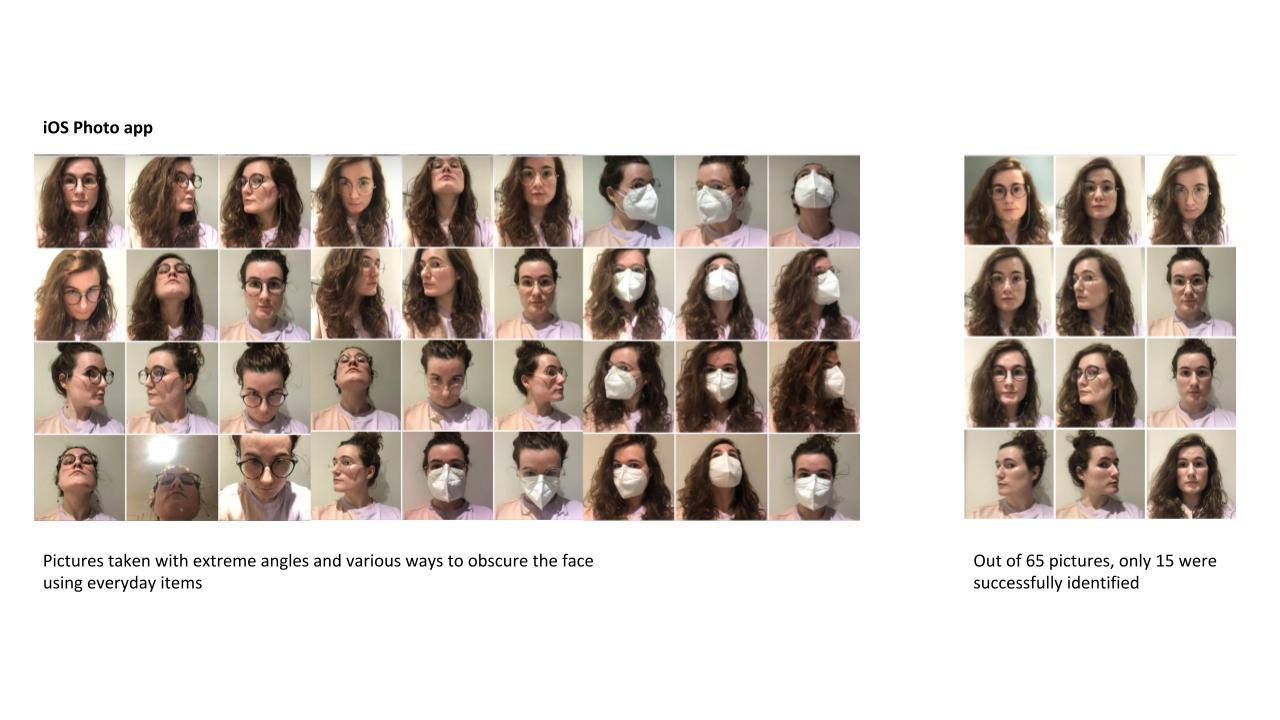
For iPhone users, scrolling to the bottom of your Albums folder will show an interesting sorting option: “People”. The Photo app constantly tries to associate faces that seem to belong to the same person, and will give you the option to select (or remove) photos it perceives as being one person. Interacting with this option trains the application to identify the individual more accurately in the future.
In a time were people are taking a tremendous amount of photographs, of themselves and of others, these databases are becoming more relevant in understanding how machine learning and facial recognition software work. We had, like most people, over 6 years of photos accumulated on our Cloud; as such, our phones so an eerily good job at identifying recurring faces and struggles more with random people or children growing up.
As is expected, the Photo app on iOs is able to positively identify our unobscured faces, regardless of how the hair is done; however, does its accuracy change when accessories are worn?
To limit as many outside variables as possible we posed in front of a neutral background (namely a white wall), wearing the same outfit and at the same time of the day. We then took multiple sets of pictures: wearing black glasses, wearing golden glasses, no glasses. For each of these three sets, we took pictures from the front, but also turning the head as to obscure one eye, and with exaggerated top and bottom views. We also took a second series of said pictures wearing a mask, resulting in a total of sixty-five pictures.
We then headed to the People Album, to see how many versions of the face the Photo App was able to correctly identify. Out of the sixty-five, only fifteen pictures made it to the folder, mostly front-facing and looking to the right, and none of them were of us with the face mask or looking up or down.
Our results suggest that most of the pictures that were positively identified were similar to those already found in its database - particularly the angle with which the photographs were taken. The application failed to identify the faces obscured by a mask and more surprisingly, continued to have difficulty identifying the face when it was only partially obscured - when two strands of hair went over the eyes. In a time when masks are ubiquitous due to the pandemic, it is interesting to see how facial recognition softwares will be developed to certify faces that are covered up.


We followed a similar technique with Windows 10 Photo app, which also has a People folder. We had to train it beforehand, taking pictures of our face under various angles and lights and tagging ourselves until it was able to positively identify us. But instead of masks, we decided to use the CV Dazzle technique to conceal our face.
We noticed that unless the colours contrasted strongly with our skin tone, the makeup was not successful in disrupting the ability of the software in positively identifying our faces. The facial recognition software began to have difficulties when major features of the face were obstructed or changed drastically: putting foundation over the lips to remove pigmentation contrasts, darkening the T-region of the face, and adding black eyeshadow around one eye (to create asymmetry) all seemed to disrupt the capacity to recognize a face. This is in line with what we saw while using Snapchat and Instagram.
Conclusion
Our research and artefact is relevant in a era where mass surveillance and protest demonstations continue to clash, and concerns about privacy are increasing in a digitalized world. Our research supports those of others who investigate the limitations of facial recognition software and what individuals can do to disrupt the surveillance algortihm: namely, obscuring the common anchorpoints that these technologies rely on (T-region, high contrast, facial symmetry).
In expanding this project further, it would be interesting to see how the recognition patterns of these software change with individuals of different skin tones: it has already been shown that facial recognition softwares have more difficulty in positively identifying Black and Brown individuals. Since both of us have fair complexions with dark facial features, providing a strong contrast for the algorithms to work with, future iterations of this experiment should include individuals with a range of skin tones and/or facial features to see if/how these algorithms diverge in recognizing and identifying a face.
Bibliography
Adjabi, I., Ouahabi, A., Benzaoui, A., & Taleb-Ahmed, A. (2020). Past, Present and Future of Face Recognition: A Review. Electronics, 9(8), 1-52. Retrieved from https://www.mdpi.com/2079-9292/9/8/1188/htm
Bacchini, F. & Lorusso, L. (2010). Race, again: how face recognition technology reinforces racial discrimination. Journal of Information, Communication & Ethics in Society, 17(3). 321-335. Retrieved from http://dx.doi.org.gold.idm.oclc.org/10.1108/JICES-05-2018-0050
Benjamin, R. (2019). Race after Technology. Massachusetts, USA: Polity Press
Bueno, C. C. (2019). The Face Revisited: Using Deleuze and Guattari to Explore the Politics of Algorithmic Face Recognition. Theory, Culture and Society, 37(1), 73-90. Retrieved from https://doi-org.gold.idm.oclc.org/10.1177/0263276419867752
Buolamwini, J. & Gebru, T. Gender Shades: Intersectional Accuracy Disparities in Commercial Gender Classification. Proceedings of Machine Learning Research, 81, 1-15. Retrieved from http://proceedings.mlr.press/v81/buolamwini18a/buolamwini18a.pdf
Fussey, P. & Murray, D. (2019). Independent report on the London Metropolitan Police Service’s trial of Live Facial Recognition technology. The Human Rights, Big Data and Technology Project, 20-22. Retrieved from https://48ba3m4eh2bf2sksp43rq8kk-wpengine.netdna-ssl.com/wp-content/uploads/2019/07/London-Met-Police-Trial-of-Facial-Recognition-Tech-Report.pdf
Lamb, H. (2020, Sept. 4). Facial recognition for remote ID verification wins Africa Prize. E&T, Engineering and Technology. https://eandt.theiet.org/content/articles/2020/09/facial-recognition-for-remote-id-verification-wins-africa-prize/
Nisbet, N. (2004). Resisting Surveillance: identity and implantable microchips. Leonardo, 37(3), 210-214. Retrieved from https://doi.org/10.1162/0024094041139463
Crawford, K. & Paglen, T. (2019, Sept. 19). Excavating AI: the politics of training sets for Machine Learning. Excavating AI. Retrieved from https://excavating.ai
Duggan, B. (2014, Oct. 22).Performance Art and Modern Political Protest. Big Think. https://bigthink.com/Picture-This/performance-art-and-modern-political-protest
Monahan, T. (2015). The right to hide? Anti-surveillance camouflage and the aestheticization of resistance. Communication and Critical/Cultural Studies, 12(2), 159‑178. https://doi.org/10.1080/14791420.2015.1006646
McGarry, A., Erhart, I., Eslen-Ziya, H., Jenzen, O., & Korkut, U. (Éds.). (2019). The aesthetics of global protest : Visual culture and communication. Amsterdam University Press. https://doi.org/10.2307/j.ctvswx8bm